How do artificial and human intelligence differ? Why does research of the subconscious matter when dividing work between robots and humans? Should VTT build autonomous super AI?
Rick Deckard, the main character in the film, Bladerunner, kills replicants – machines that resemble humans – for a living. However, by the end of the film, Deckard, who is played by Harrison Ford, falls in love with a replicant. Deckard’s ambivalence towards replicants reflects the current debate about artificial intelligence. Some predict huge changes and see super AI plotting to take control, in a very human manner. Others are more unconvinced: ‘Replicants are like any other machine,’ said Deckard as well, before he changed his mind. Machines modelled on people is therefore a classic science fiction idea. I want to enter the debate by comparing human and artificial intelligence. I discuss the argument that it is difficult to copy human intelligence in machines, because our intelligence cannot be separated from its environment. In addition, I would claim that analysis of human activity would be useful when remodelling working life.
Human intelligence
Conscious language-based thinking (inner conversations) is just the tip of the human intelligence iceberg. When an expert is asked how they managed to solve a problem or conflicting situation, they often answer that they ‘just knew’. Human expertise is a combination of schooling and book learning, personal conscious thinking, and something based on learning by doing. A knack and feeling for something, an expert eye and ear, and vision are popular ways of describing the tacit knowledge and ‘feel’ we have for performing various tasks. A person works intuitively and adaptively, selectively using millions or perhaps billions of sensory cells, depending on the situation.
It could be argued that intelligence and knowledge are located in the connections between the brain and countless sensory cells and nerves, rather than simply in the brain. Experimental psychology has shown that, in many respects, human activity and decision-making are directly connected to the environment. Action does not therefore require conscious thinking. The idea that our thought processes are embedded in our experienced environment is logical, since cerebral intelligence is connected to the sensory cells, which are themselves in direct contact with the environment.
In addition to having bodily intelligence, people are able to interpret and learn meanings. We excel at this in comparison to other species, due to the way in which our ancestors gathered food. Over short distances, humans are often outpaced by their prey, but we can jog for extremely long distances. Game therefore had to be followed over a very long journey. This was done by following tracks. We interpreted signs imprinted in the environment in order to survive – reading is species-typical behaviour for us. Now also people read continuously; some read their mobile phones, while others read newspapers. Because our senses are relatively dull, we cannot identify poisonous plants and fungi from the edibles just by smell, but by learning to distinguish the good from the bad. That is why adults are also generally able to distinguish good from bad, in other words we have a moral conscience. It is highly apt that Adam and Eve ate from the tree of knowledge, of good and evil, in the Book of Genesis. Conscious thinking was essential in the everyday lives of hunter-gatherers. People paid a price for stupidity even then – our intelligence and consciousness are by-products of evolution.
Artificial intelligence
Artificial Intelligence makes predictions on the basis of data and follows written instructions. Its predictive capabilities are based on neural networking in particular. Neural networks consist of mathematically interconnected nodes, i.e., neurons. Initially, the connections are typically random, but they are then strengthened and weakened through trial and error. For example, thousands of images, categorised in a manner meaningful to people, can be fed into a neural network: ‘woman’, ‘man’, ‘cat’, ‘dog’, etc. By grouping certain features, the neural network can guess what each image contains. The right answers reinforce the pathways between network nodes that lead to the right answers. Pathways that lead to the wrong answers are weakened. This ‘guessing machine’ becomes an effective predictor after hundreds of thousands of tries. Prediction is ultimately so certain that artificial intelligence can identify images or words from a soundtrack.
Many kinds of human activity can be performed through prediction and by following instructions. We may wonder, for example, whether artificial intelligence is capable of creative tasks. AI can be made to compose musical pieces: it can analyse the melodies of the most popular compositions and predict the most catchy ones on that basis. With very careful preparation, it could also stretch to writing some lyrics and instrumentation. But music is more than sounds: young people have a tendency to develop their own, original vibes and grooves, which irritate their elders. AI cannot truly create new musical genres that reflect the times that the listeners are living through. Music cannot be separated from dance styles and the issues we consider important. And which is the creative actor here – artificial intelligence or the software developer?
Artificial general intelligence (or AGI) refers to AI capable of design, adaptation, reasoning and linguistic communication in a similar manner to humans. Definitions and proposed criteria for AGI tend to vary. A machine succeeding in making coffee in a strange flat could be a sign that we are in the presence of AGI. In the scientific community, there is no consensus on whether AGI is even possible in principle. Both Microsoft and Google are running research programmes to achieve AGI. This is understandable, because sceptics rarely find their way into top positions in American blue chip companies. These programmes will undoubtedly lead to commercially exploitable technologies, even if they do not actually achieve AGI itself.
Cognitive architecture and task analysis
In principle, to build a truly human robot, we need to analyse people themselves. Cognitive scientists use the concept of cognitive architecture to describe human thinking holistically, including feelings and other matters. Because people are so fiendishly complex, it seems to me that the study of general cognitive architecture is better suited to basic research of artificial intelligence than the development of AI in practice.
Technology companies can meet their needs through task analysis. When replacing an employee with robots, we need to analyse what kind of work people are doing. This will ensure that the new robotised approach provides a result of at least the same quality and safety as the traditional way of operating. Since people will always be needed, even based on the new operating model, a division of labour between people and robots will be designed. Task analysis could also indicate that there is no point in robotisation.
There are many forms of task analysis. Cognitive task analysis involves modelling an employee’s thinking. Dozens of tools are available for this. Task analysis can also analyse an employee’s movements or the features of a work organisation. In particular, VTT uses the core task analysis method developed by now-retired research professor Leena Norros. The idea is to contrast observed working practices and challenges with the general goals and critical phases of work. The personal characteristics of employees are secondary: as ‘core’ suggests, this concerns the analysis of a task’s core features. Bearing the main goals in mind enables us to view a task’s performance from a number of perspectives – the same general goal can be achieved by human action or a robot. This makes core task analysis ideal as a design aid: it guides but does not cramp the designer’s creativity. Depending on the research questions, core task analysis can flexibly include various features of cognitive task analysis, the micro-level analysis of work practices, or the modelling of the operating environment.
It is beneficial to blur the boundary between worker and researcher when mapping cognitive processes during task analysis. Workers are seldom aware of their own mental models while working, because skills are subconscious in nature and the subconscious cannot be directly studied by transferring information from the employee to a researcher. The idea is that the researcher and worker together explore issues hidden in the subconscious, which means that the employee, in a sense, becomes both ‘teacher’ and ‘pupil’ at the same time. Workers love task analysis of this kind, because they find insights about their own work fascinating by nature. A good, practical technique for this involves watching a video of the worker in action, together with him or her.
User-centred AI research
Human and artificial intelligence are different. Even an accurate study of people will not enable us to create actors that precisely resemble humans. The quantum computers of the future may be millions of times faster than today’s computers. However, this does not alter the fact that, in the future, artificial intelligence will not be able to function adaptively in the real world as people do. In the absence of new developments, we would still fail to achieve seamless interaction between the complex whole of the sensory system and decision-making. As concepts, neural networks and artificial intelligence hint at the replication of human intelligence. However, in practice it would make more sense to study the use of AI.
Without research, it is hard to figure out how different work assignments may benefit from data-based predictions. Through object-recognition capabilities, AI will solve problems in a number of job tasks, because camera technology is already used for other purposes in many sectors. Surgeons, for example, often use a camera image when operating: in the future, AI may help to identify cancer cells and nerve paths. It is to be explored how work-life focused user research could provide practical tools for the building of neural networks. Interdisciplinary work is necessary.
The author is exploring the division of labour between AI and people in a project that explores the future of seafaring. He has performed task analysis blurring the boundaries between worker and researcher in Academy of Finland project called WOBLE, which he led, focusing on robot-assisted surgery.
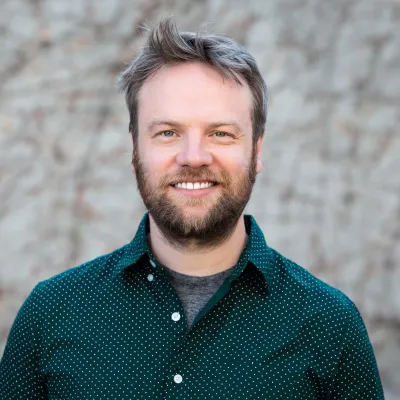